Generative AI in Product Design
My new ebook offers case studies on AI and just enough theory for you to build your next app with gen AI.
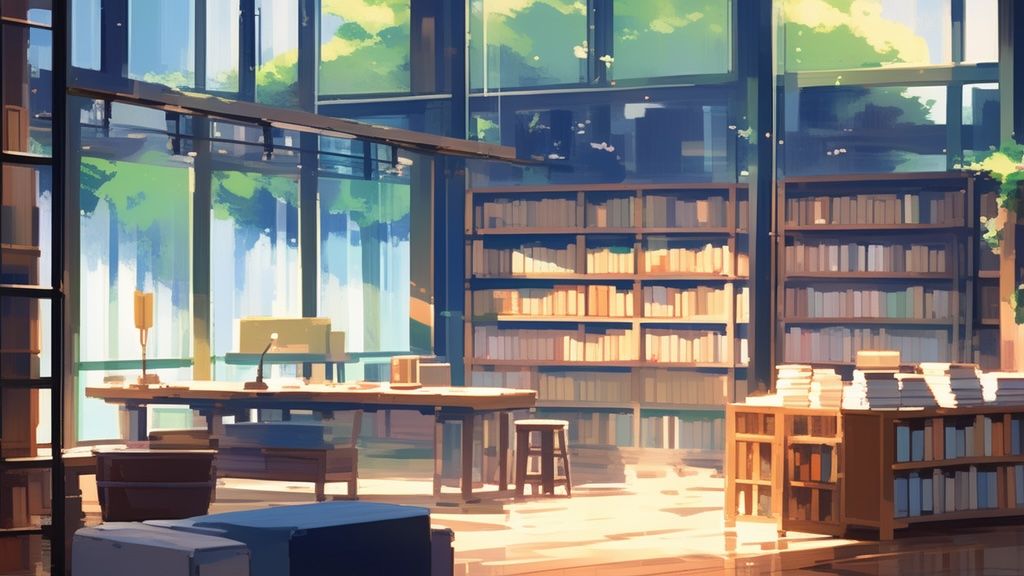
You may have noticed that in recent months, Before Growth has alternated between AI and other early-stage startups. In a year in which AI startups made up over 60% of Y Combinator’s startup class, this was deliberate. But there was also another reason behind this choice.
When I began structuring my ideas about AI, it became clear that I had more material than what a few newsletter issues could cover. In fact, the content was extensive enough to fill a short book. This led me to think: Why not write it? And that’s how Generative AI in Product Design, an ebook focused on designing and building products in the AI era, came into this world.
The ebook combines fresh content and familiar materials from Before Growth, presented in a more structured manner. Currently, it includes three chapters, the details of which and the complete table of contents are provided below. Spanning 74 pages, it offers valuable insights, engaging case studies on AI startups, and just the right amount of theory to equip you for developing your next app with generative AI.
If you want to get into the early-access program, subscribe to Premium to become a paid member. Also, all subscribers will get the finished version when it’s done—but I’m not yet sure when that will be.
For a clearer idea of the book’s content, I’m sharing a free preview of selected parts from Chapters 1 and 2 below.
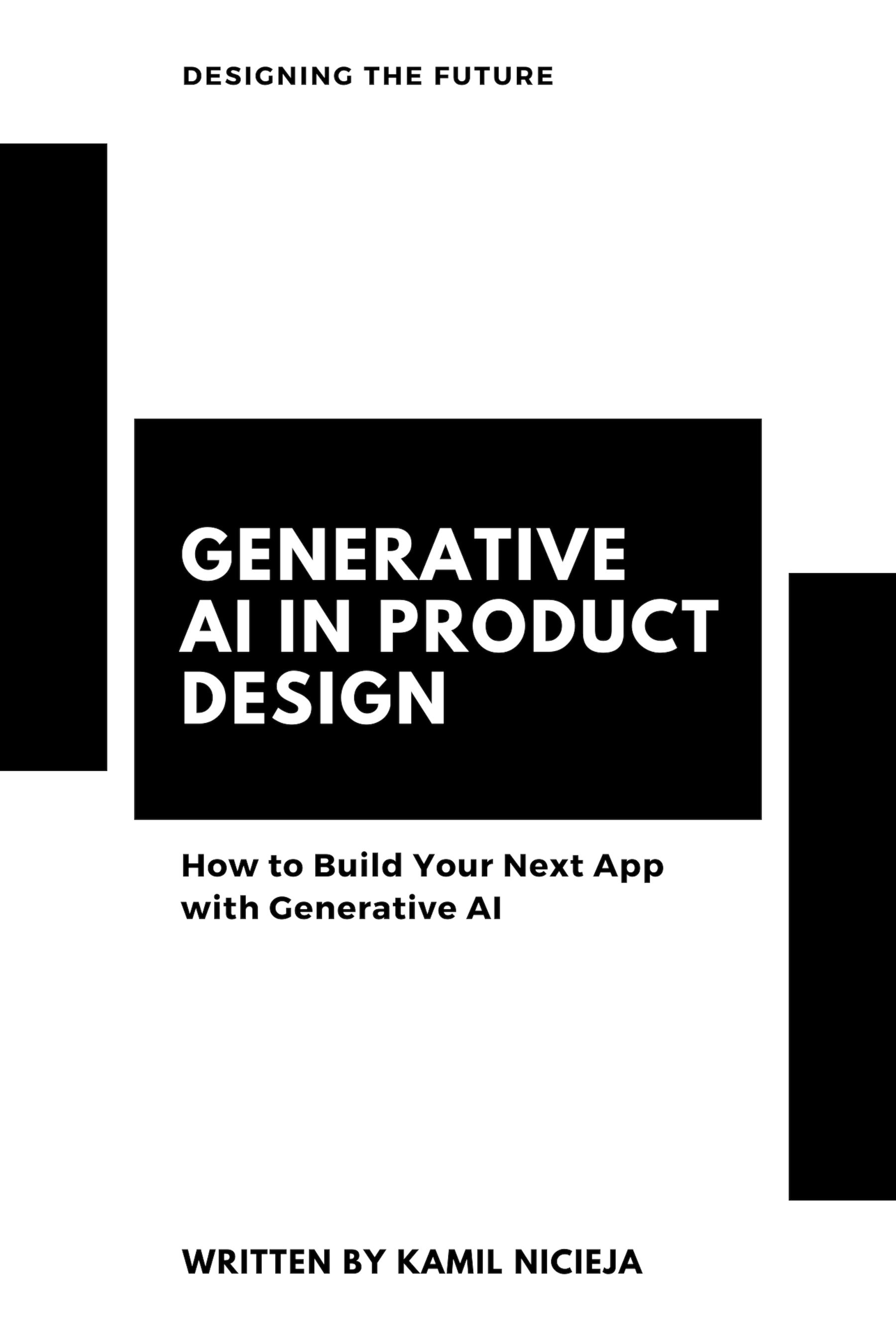
About this book
The goal of this book is to equip you with the skills to:
- Grasp the fundamentals of generative AI based on practical case studies with just the right amount of theory
- Incorporate gen AI methods into your product design efforts
- Create new applications, ventures, and startups using generative AI technologies such as OpenAI’s GPT–4, DALL–E, or open–source alternatives like Llama and Stable Diffusion
- Hone your ability to effectively prompt these models
- Navigate the complete journey of crafting products powered by generative AI, from budget allocation and design to selecting between in–house and third–party solutions, and then to building prototypes
In Chapter 1, we explore the vast potential of generative AI in revolutionizing various industries. Chapter 2 talks about the nooks and crannies of prompting, highlighting its role in creating a new wave of products highly responsive to human interaction. However, generative AI is still emerging in terms of widespread adoption. While promising, the technology is not without its hurdles and constraints. It isn’t all–powerful.
In Chapter 3, we will address challenges that product professionals need to overcome for effective use of generative AI. We’ll begin with technical constraints, one of which—hallucinations—you’ll also get familiar with in Chapter 2. There are other similar challenges to explore. Next, we will transition to issues tied to product and design before concluding with obstacles associated with AI–driven business strategies and potential legal concerns.
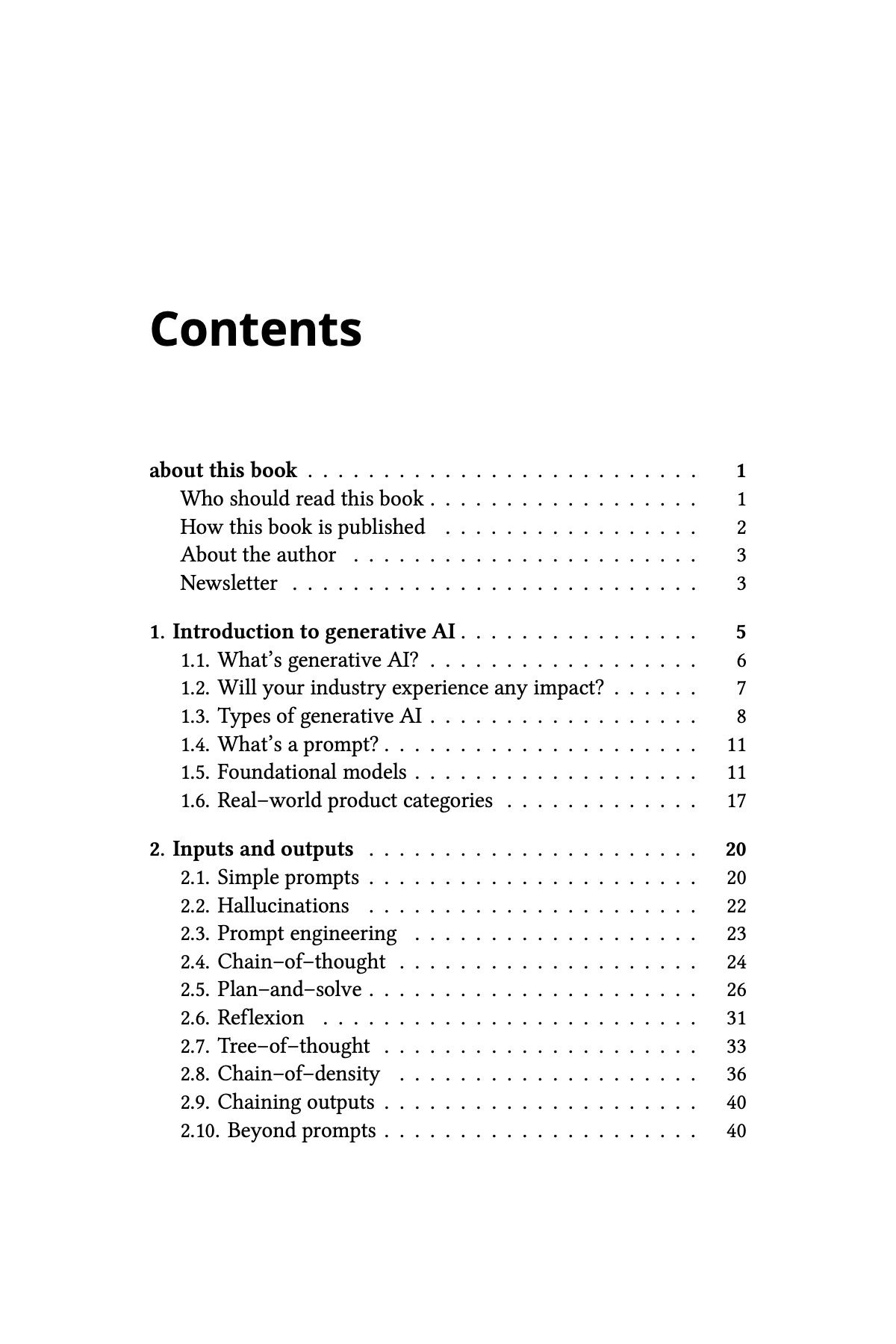
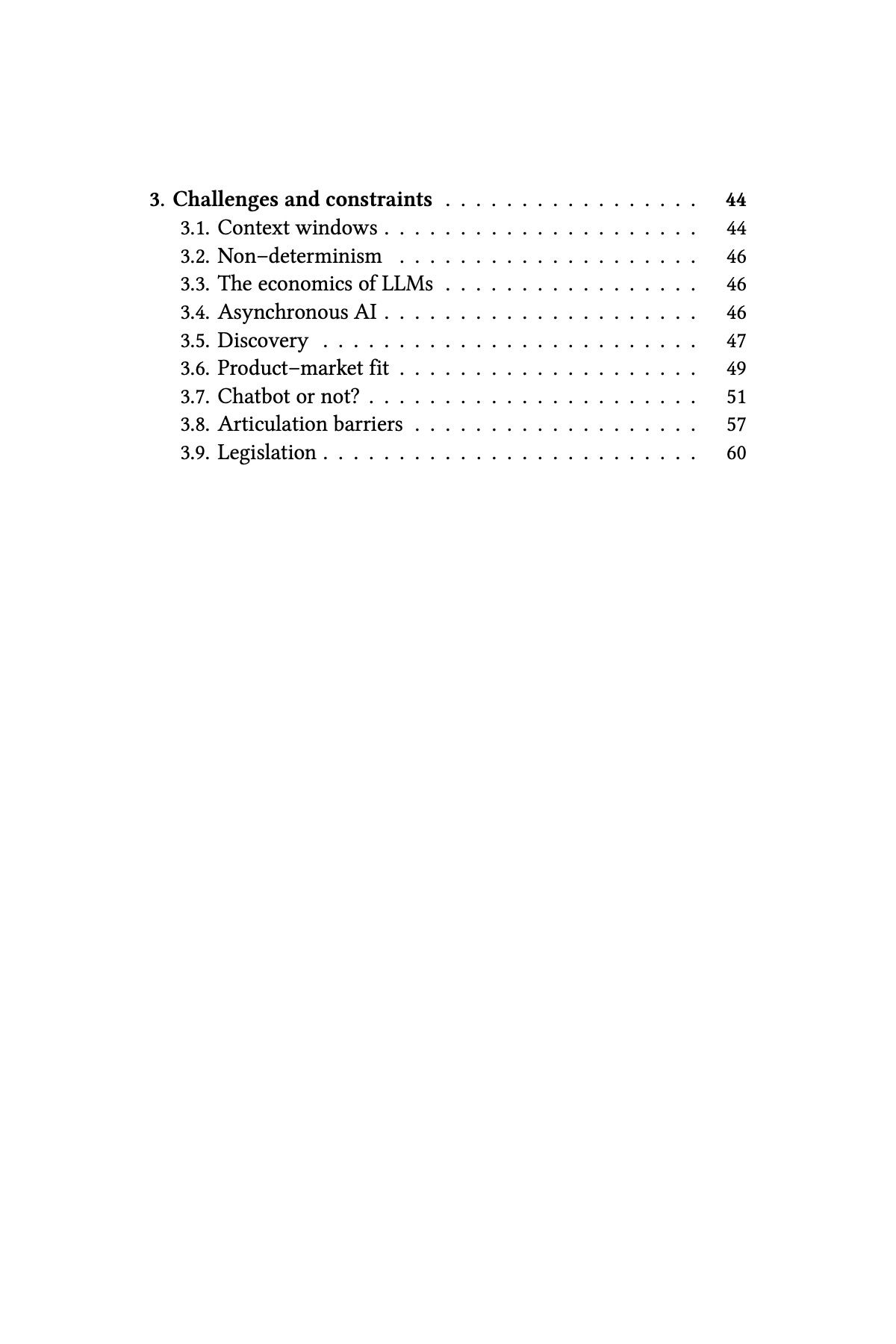
Table of contents
Who should read this book
This book is intended for intermediate–level readers eager to apply generative AI models, particularly in the realm of new product development. When I refer to “product design,” I’m talking about more than just the user experience or user interface. I mean the comprehensive, high–level process of developing a product from start to finish. Keep in mind: design isn’t just what something looks and feels like—the design is how it works.
While this book is technical in nature, coding skills aren’t a prerequisite for grasping its content. It’s tailored for professionals—be they engineers, designers, project managers, executives, or founders—who have already brought products to market and are now seeking an introductory guide to integrating generative AI into their process.
Instead of focusing too much on technical challenges, this book maintains a high–level perspective. This approach allows non–engineers to understand how they can meaningfully contribute to their team’s AI initiatives without necessarily running code themselves. For those with technical expertise, the book offers insights into practical applications of their deep knowledge of AI model internals, especially when it comes to developing and launching new applications.
Chapter 1. Introduction to generative AI
When it comes to AI, we’re still in the early days. The majority of people haven’t yet adopted these tools for their work, hobbies, or day–to–day lives.
Some have experimented with early versions and found them lacking, abandoning ship before the current wave of tools, with their significantly improved capabilities, came into wider use. Even those who have found some applications often don’t venture beyond their starting point, opting to use these models for basic outputs.
Just recently, I assisted a friend who runs a one–person business with employing AI as a virtual marketing consultant. We used the tool to generate content and strategic ideas for the company. Yet, like all things, the assistant had its limitations. It needed many guiding questions and iterative refinements to produce truly valuable output. If you just skim the surface, it will do so, too. But the outcomes were still notably better than what you’d expect from someone with no marketing experience.
And while I believe the term “prompt engineering,” which we’ll talk about in the later chapters, is overhyped, I do think a particular mindset is necessary when working with neural models: you’ve got to guide and steer them. It’s an iterative refinement process, and anything less will yield rather superficial results—at least as things stand now.
So, if you’re anxiously searching for an avenue to break into the AI field, concerned you’re already behind due to the progress of others, here’s a tip: learn to prompt well and then help somebody who can’t. As you can probably guess—this book is my way of doing so.
Will your industry experience any impact?
Every industry will experience generative AI’s impact. The only question is how much.
Obvious examples include industries like marketing, which could use generative AI for creating ad copy, social media posts, or even for strategy planning. In the legal industry, AI could help in drafting legal documents or contracts based on a set of user–defined parameters. This would speed up many administrative tasks, allowing lawyers to devote more time to complex legal issues. In HR, AI could assist in the initial stages of candidate screening by generating interview questions based on the specific needs and culture of the company, or even by assessing the suitability of applicants through automated analysis of resumes and cover letters.
What might this actually look like in a real–world scenario? Let’s walk through a brief case study to visualize some concrete effects.
The gaming industry stands to gain significantly from generative AI, too. We can think of an extreme example: a game that’s entirely customized for each player’s unique experience, generating assets on–the–spot. This could involve creating a personalized narrative, textures, and dialogue based on the player’s decisions, thereby offering a completely non–linear gaming experience. However, it’s probably safe to say that we’re still many, many years away from being able to pull something like this off on a AAA scale.
But what about indie games? Take Jussi Kemppainen from Dinosaurs Are Better as an example. He’s single–handedly developing an entire adventure game, using AI assistance in all aspects of game design, ranging from character creation to coding, dialogue crafting to graphic design.
Let’s consider another application.
Imagine a detective game where you play as a police investigator trying to solve a murder. Your task is to interview numerous witnesses and suspects to discover the true culprit. Each character you interact with would be powered by a sophisticated model, each embodying a specific persona. These personas would have two stories: the one they willingly share with investigators, and the hidden truth that they’d prefer to keep secret for various reasons. As the player, your goal would be to interrogate each persona, gradually unraveling the truth to identify the real offender. To add an extra layer of complexity, we could introduce a judge persona. Instead of merely choosing who they think is guilty, players would need to build and present a compelling case to the judge, effectively discouraging random guesswork.
The user interface for such a game could be as simple as a chat window, with the main gameplay focusing primarily on dialogue–based interaction. I believe it’s feasible to create a prototype of such a game with our current technology. This could serve as a powerful proof of concept for the kind of non–linear, immersive experiences generative AI could eventually enable in the gaming world.
I trust these examples will ignite your imagination, inspiring you to consider how these AI models could reshape your field—even right now, let alone what they might achieve in the next five to ten years.
What’s a prompt?
Prompts serve as the input queries that guide these models to generate a specific type of output. The function of a prompt varies slightly between these two kinds of generative models, but the core principle remains consistent: a prompt initiates the generation process and steers the model toward producing content that aligns with the user’s intention.
For large language models, prompts are typically text–based queries or statements. They can range from simple requests, such as “Tell me about the history of Rome,” to more complex or conditional queries, like “Write a persuasive essay arguing for renewable energy adoption.” The prompt is fed into the model, which then produces text that ideally satisfies the query, all while adhering to the grammar, tone, and context specified.
In the case of text–to–image models, prompts still serve as input queries, but the output is visual rather than textual. Here, the text–based prompts may include descriptions or features that the user wants to see in the generated image. For example, a prompt like “a serene beach at sunset” would guide the model to generate an image of a beach with qualities that could be described as serene and with lighting conditions consistent with a sunset.
Naturally, various models yield distinct outcomes, but prompts always shape the contours of the output—whether that output is a block of text or a visual image.
Foundational models
While these models come in many forms, they all share a common characteristic: they’re large. Exceptionally large.
The size of these models is quantified in terms of parameters. A model parameter is an internal configuration variable whose value can be derived from training data. Essential for making predictions, these parameters determine the model’s effectiveness in solving specific problems. They are learned aspects of the model, gleaned from historical training data. Parameters are fundamental to the operation of machine learning algorithms, and some of these models boast hundreds of billions of them.
The sheer scale of these models precludes individual developers or small organizations from training them, due to prohibitive computational and financial costs. As a result, they are typically developed by major tech companies or heavily–funded startups. This is why these models are often termed “foundational,” as they serve as the base upon which other applications and services are built.
We’ll explore some of the most prominent foundational models available at the time this book was written.
Which AI model should you use?
Here’s a concise overview of the top–performing models for specific tasks as of October 2023:
- Coding → GPT–4
- Brief interactions with rapid replies and minimal precision expectations → GPT–3.5
- Artsy images → Midjourney
- Personal conversations → Pi
- Self–hosted tasks → Llama
- Image analysis tasks → Bing
- Images with text → DALL–E 3
- Copywriting tasks → Claude 2
- Long context tasks → Claude 2
- Internet browsing tasks → Bard
- Internet–based sources → Perplexity
- Harder reasoning tasks → GPT–4
- Long–form writing tasks → GPT–4
- Analyze large PDFs → Claude 2
- Realistic images of humans → Adobe Firefly 2
- Data analysis → ChatGPT Advanced Data Analysis
Selecting an appropriate model involves weighing factors such as result quality for distinct tasks, cost, speed, and availability. The decision is multifaceted. Chapter 3 will dig deeper into the intricacies of LLM economics, drawing parallels and distinctions with other cloud–based services.
Real–world product categories
There are a few categories of products where these models are already being used in.
The leading category in this space is AI assistants, which have evolved significantly beyond earlier versions like Apple’s Siri or Amazon’s Alexa. The key distinction lies in how interactions are programmed. Earlier assistants relied on hand–coded responses, excelling in narrow tasks like weather reporting but falling short in complex assignments. In contrast, LLMs can easily draft a creative homework assignment, but are, in exchange, prone to factual inaccuracies due to a phenomenon known as “hallucinations.” We’ll discuss it in the next chapter. So while these models represent the most comprehensive aggregation of human knowledge to date, they lack an inherent understanding of truth.
AI assistants are typically designed for general–purpose use, capable of performing a wide range of tasks. However, a burgeoning subcategory focuses on AI companionship. These are specialized AI personas that serve as your digital friends, peers, or acquaintances. For example, apps like Character allow you to engage in conversations with characters from your favorite books and movies just for entertainment. Meanwhile, platforms like Facebook are developing AI personas intended to act as, say, your personal trainer, motivating you to hit the gym.
Another category includes AI utilities, which can be integrated either into the front end or the back end of existing products. On the front end, these utilities could include features like document summarization in platforms like Google Docs, voice transcription in Zoom, or automated thumbnail generation for WordPress articles. On the back end, large language models can be employed for tasks like optical character recognition or tagging unstructured text data at scale. While these capabilities offer substantial utility, they usually need to be incorporated into broader products to be truly effective, as they provide limited standalone value.
The most innovative yet least validated category in the realm of generative AI consists of autonomous agents. While it’s debatable whether these models can truly think like humans, they have demonstrated the ability to mimic certain aspects of human thought. For instance, they can tackle logical problems, ranging from simple to complex, and some can even ace university exams.
This has led to their use in creating task–solving loops. Essentially, you can instruct a model to devise its own action plan, then have it execute the steps it outlined. A rudimentary example would involve asking a large language model to generate a table of contents for a book, then having it create subsequent chapters based on that framework until the book is complete. While potentially revolutionary for multiple industries, these autonomous agents are still in early stages, delivering results that are too inconsistent for reliable production use.
Chapter 2. Inputs and outputs
Like many others, my interest in text–based AI was sparked when I started using Siri on my iPhone.
Siri, a chatbot developed before the advent of modern generative AI, has interactions that are predefined rather than limitless. Its capabilities, such as providing weather updates, were anticipated and manually programmed by Apple engineers. This involves querying an API for weather conditions to answer such queries.
But if you ask Siri to write a poem about a recent event, it won’t be able to assist, as its responses to such specific requests aren't predefined. It’ll probably direct you to a Google search instead. In contrast, modern AI assistants can autonomously create a poem, albeit of varying quality, by filling in the details themselves.
These questions and commands—they’re called prompts. When prompted, a large language model generates text, while a diffusion model creates images. In this chapter, we’re going to examine the types of prompts these models can handle, the underlying mechanics that drive their responses, the structure of prompts, and the ways in which they can be creatively applied in product design.
Simple prompts
Let’s begin with something straightforward. I’ve compiled a selection of good prompts I’ve used with ChatGPT in the past 30 days. If you haven’t had the chance to interact with a large language model, this list should provide you with an overview of the fundamental capabilities of models like GPT–4.
“Can you provide 10 alternative names for this piece of code?” This could be for a method, variable, or constant.
“I’m not a native English speaker. Can you help me rewrite this sentence or paragraph to sound more like one?” Sometimes I’m just fine with sacrificing some of my personal voice for the sake of clearer and more professional English.
I often request translations, too. GPT–4 has already proven to be much more accurate and reliable than Google Translate in this aspect.
“Could you interpret this code for me? I’d appreciate a step–by–step explanation.” If the explanation is unclear, then it’s likely that my fellow engineers might also find it hard to understand, indicating a need for refactoring.
“Let’s discuss the new feature I’m developing. I’d like you to act as a team member and review my proposed implementation method step by step.” Alternatively: “I’m faced with a particular issue or feature to be developed. Could you suggest how you might approach it?” This is particularly helpful when I hit a creative roadblock.
Conventionality checks. ChatGPT typically defaults to a safe, conventional response. This can be limiting when looking for creative inputs, but in certain contexts, such as some engineering decisions, a conventional response might be ideal as it’s likely to be more widely understood.
Pros and cons. Ask ChatGPT for arguments in favor of and against a certain concept. The responses can help gauge how your thoughts align with or deviate from common viewpoints.
Finding synonyms. With ChatGPT readily available, it’s quicker to ask it for synonyms than to look them up in a dictionary.
Tackling anything I’m below average at. Take, for instance, naming characters in the short stories I write. I used to struggle with this, so now I ask ChatGPT for similar character names based on those I like. Even if none of the suggestions strike a chord, I can ask for the reasoning behind each name and use that as a springboard for further ideas.
“I need to write a performance review, create a blog article, or draft a long email, and so on—but I’m finding it hard to start. Can you ask me questions about the person or topic until we’ve gathered enough information to form a comprehensive review?” This approach helps overcome writer’s block, as conversations with ChatGPT are informal and free–flowing.
We can identify some basic patterns in these prompts. As a programmer, I often use ChatGPT like a peer to help solve coding problems. I also write a lot, so it serves as a writing partner for me. This approach reflects how many others interact with these models and the trend in early modern AI products. They were job–focused, leading to the emergence of AI roles like programmers, lawyers, security consultants, writers, and more.
Hallucinations
So, does that mean the task is complete? Just slap AI on every white–collar job and voilà, a fresh product idea emerges, and product design becomes a solved discipline. Well, not exactly. Despite their skill, these models are not all–powerful.
Because they hallucinate.
Hallucinations in large language models refer to instances where the output is coherent and grammatically correct, yet factually inaccurate or nonsensical. In this context, a “hallucination” is the creation of false or misleading information. Such errors can emerge from several causes, including limited training data, biases within the model, or the complex nature of language itself.
For example, in February 2023, Google’s chatbot, Bard, mistakenly stated that the James Webb Space Telescope captured the first image of a planet outside our solar system. This was inaccurate; NASA confirmed that the first images of an exoplanet were actually captured in 2004. Furthermore, the James Webb Space Telescope wasn’t launched until 2021.
And in June 2023, there was a case where a New York attorney used ChatGPT to draft a motion that contained fabricated judicial opinions and legal citations. The attorney faced sanctions and fines, claiming that he was unaware that ChatGPT had the capability to generate fictitious legal cases.
The key issue with hallucinations is that they are not merely a glitch; they are inherent to the design. These models are generative in nature. Without the ability to deviate from their training data, they would be restricted to replicating what they’ve previously encountered, limiting their usefulness. They would be reduced from reasoning tools to mere search algorithms—a problem search engines like Google have already addressed. Fundamentally, these models don’t possess definitive answers to the questions posed to them. Their core function is to predict the next word based on probabilities. When given a prompt, they identify the most likely word sequence that resembles responses to similar inputs in their datasets.
Prompt engineering
Is it possible to solve the problem of hallucinations in generative AI models? And if so, how? Considering the probabilistic nature of these models, complete eradication of hallucinations isn’t feasible. However, there are developed techniques to mitigate the issue. Since LLMs are trained for next–word prediction, the solution partly lies in the formulation of the question itself. By refining our prompts or questions, we can achieve more accurate and reliable outcomes.
That’s the reason behind the emergence of prompt engineering. This new field focuses on crafting and optimizing prompts to maximize the efficiency of language models across diverse applications and research areas. Developers engage in prompt engineering to create strong and effective techniques for interfacing with LLMs and other tools, aiming to minimize issues like hallucinations.
To grasp this concept in action, we’ll analyze advanced prompting methods like chain–of–thought, tree–of–thought, and reflexion. These techniques enhance the model’s ability for deeper and more nuanced reasoning, narrowing the gap between user expectations and the model’s actual capabilities. When building AI–powered products, engineered prompts are likely to be the ones you’ll predominantly use. The simpler prompts previously mentioned? They might work in B2C apps. But for B2B solutions? Forget it. Given the high stakes, would you risk having your enterprise product generate unreliable outputs? Yeah, I thought so.
Chain–of–thought
Let’s start with chain–of–thought. This is a novel strategy that prompts the language model to articulate its thought process. By exposing the model to a handful of examples where the reasoning is clearly spelled out, the model learns to similarly outline its reasoning when responding to new prompts.
To illustrate a chain–of–thought approach using different examples than those provided, let’s consider a scenario involving a bookstore and another involving a school’s sports equipment inventory.
Model input:
Q: A bookstore has 200 novels in stock. It receives 4 more boxes of novels, each box containing 25 novels. How many novels are in stock now?
A: Initially, the bookstore has 200 novels. Each of the 4 boxes contains 25 novels, so 4 boxes total 4 × 25 = 100 novels. Adding these to the original stock, 200 novels + 100 novels = 300 novels. The answer is 300 novels.
Q: The school’s sports department has 15 basketballs and 10 volleyballs. If they purchase 3 new sets of sports equipment, each set containing 5 basketballs and 4 volleyballs, how many of each type of ball do they have now?
Model output:
A: The sports department starts with 15 basketballs and 10 volleyballs. Each set of new equipment contains 5 basketballs and 4 volleyballs. So, 3 sets would have 3 × 5 = 15 basketballs and 3 × 4 = 12 volleyballs. Adding these to the original numbers, the department now has 15 + 15 = 30 basketballs and 10 + 12 = 22 volleyballs. The answer is 30 basketballs and 22 volleyballs.
As models advanced in intelligence, it became less crucial to provide examples of reasoning. The training datasets already contain a vast array of reasoning examples! Depending on the size of the model, you can activate chain–of–thought processing in almost any prompt by using a magic phrase: “Let’s think step by step.”
Model input:
Q: The school’s sports department has 15 basketballs and 10 volleyballs. If they purchase 3 new sets of sports equipment, each set containing 5 basketballs and 4 volleyballs, how many of each type of ball do they have now? Let's think step by step.
Model output:
A: The sports department starts with 15 basketballs and 10 volleyballs. Each set of new equipment contains 5 basketballs and 4 volleyballs. So, 3 sets would have 3 × 5 = 15 basketballs and 3 × 4 = 12 volleyballs. Adding these to the original numbers, the department now has 15 + 15 = 30 basketballs and 10 + 12 = 22 volleyballs. The answer is 30 basketballs and 22 volleyballs.
By outlining the thought process, the AI’s responses become more transparent and easier to inspect. Users can see how the AI arrived at its conclusion, which helps in verifying the accuracy and reliability of the response. By seeing the intermediate steps in the AI’s reasoning, users can more easily identify where the LLM might have gone wrong and provide targeted feedback for improvement.
For tasks that require sequential steps or reasoning, chain–of–thought prompting can guide the AI to follow a structured approach, leading to more accurate and relevant answers. When the AI explains its reasoning step–by–step, it can also be an educational tool, helping users learn how to approach similar problems in the future.
Case study: The smart reactivity of LLM–based apps
You might be wondering: This is nice and all, but how can I apply what I’ve learned? Am I supposed to create a calculator app? That’s not my goal! And I would agree with you. Now that we’ve grasped this piece of theory, let’s apply it to a real, though not overly complex, project.
There’s a limit to what you can achieve using just tags, keywords, likes, votes, and other “simple” metadata.
The first wave of metadata–driven products emerged with the advent of social networks. Platforms encouraged users to “like” various items and operated on the naive assumption that if individuals within your network appreciated something, you would likely enjoy it as well. And so we got Digg, Facebook, Twitter, YouTube, and many, many more…
The second generation of smarter reactivity leveraged classification algorithms. Consider TikTok, for example. It cleverly employs AI to determine the content you engage with, then curates more of what might appeal to you, bypassing your social connections. Just watch the stuff you like—we’ll figure out the rest on our own. While this was groundbreaking at a large scale, it’s only scratching the surface of what’s next.
Enter large language models.
The upcoming wave will pivot from mere classification to reasoning and cognition. Even though LLMs sometimes err and glitch, they exhibit a semblance of reasoning in many straightforward scenarios. The debate on whether this mirrors human–level thought is ongoing, but for many applications, even current capabilities suffice. Let me illustrate with a personal example.
As a proof of concept, I built Changepack, an open–source changelog tool integrated with ChatGPT. Changepack syncs with your GitHub activity, streamlining progress tracking. Every month, Changepack selects the most noteworthy updates, crafting a release note draft for your perusal and dissemination.
This selection process harnesses ChatGPT. In essence, I task the algorithm with sifting through recent changes, selecting the most pertinent ones, and justifying its choices for optimized outcomes. This allows me to proactively compose a draft for release notes without any human input, compelling the AI to scrutinize the core content, and deduce conclusions for me, sidestepping the need for behavior–based metadata.
This process involves two steps. Initially, AI needs to assess each change:
(…)
If you want to get into the early-access program, subscribe to Premium to become a paid member. Also, all subscribers will get the finished version when it’s done—but I’m not yet sure when that will be.
This post is for paid subscribers
Ready to stay ahead of the curve? This post offers just a taste. Unlock the full experience and gain access to hand-picked tech industry intel. Don’t miss out on the ideas that will shape the next decade—subscribe to Before Growth now.
SubscribeAlready have an account? Log in